How AI is improving fraud detection in banking systems
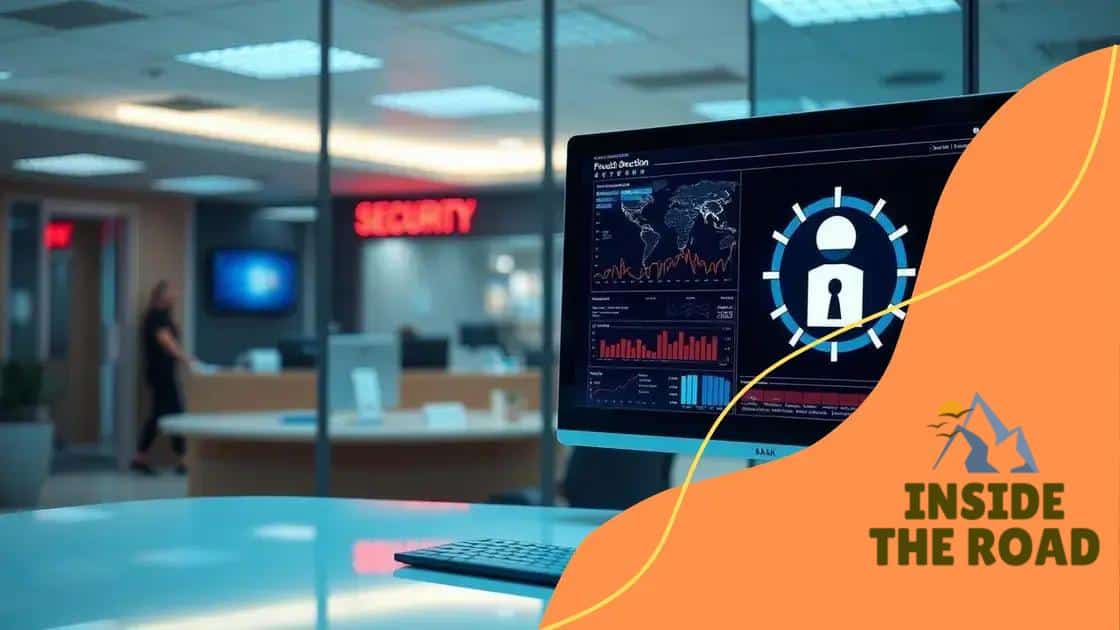
AI is significantly improving fraud detection in banking systems by utilizing advanced algorithms that analyze transaction data in real time, predict potential fraud, and enhance security through biometric measures and collaborative efforts among financial institutions.
How AI is improving fraud detection in banking systems is a game changer for financial institutions. With increasing cyber threats, the use of AI tools helps banks identify fraudulent activities swiftly, saving time and resources. Let’s dive into how these technologies make a difference.
Understanding AI in fraud detection
Understanding AI in fraud detection is essential in today’s banking environment. Financial institutions face serious threats from fraud, affecting their integrity and customer trust. AI technologies provide solutions to these issues, helping banks enhance security measures effectively.
Machine learning, a subset of AI, plays a crucial role in detecting irregularities in transactional patterns. By analyzing vast amounts of data, these systems can identify suspicious activities much faster than traditional methods.
How AI Identifies Fraud
The process begins with data collection. AI systems gather information from various sources such as transaction history, customer behavior, and external fraud trends. These systems then use algorithms to detect anomalies. Here are some key steps:
- Data aggregation: Combining different data points for analysis.
- Pattern recognition: Identifying typical behavior to spot deviations.
- Risk scoring: Assigning a risk level to each transaction.
- Real-time alerts: Notifying banks immediately about potential fraud.
This dynamic ability allows banks to act swiftly, reducing the number of successful fraud attempts. By leveraging vast datasets, AI can continuously learn and adapt to new fraudulent techniques.
The Benefits of AI in Banking
Integrating AI into fraud detection offers numerous advantages. Firstly, it enhances efficiency, allowing banks to handle more transactions while maintaining vigilance. Secondly, it reduces false positives, meaning legitimate transactions are less likely to be flagged as fraud. This is vital for maintaining customer satisfaction.
Moreover, AI systems can work around the clock without fatigue, constantly monitoring transactions. As cybercriminals devise new tactics, AI continues to evolve, staying one step ahead through its learning capabilities.
Benefits of AI-driven fraud prevention
Benefits of AI-driven fraud prevention enhance the security landscape in banking. By implementing AI technologies, banks can significantly reduce fraud-related losses while improving service quality for customers. The effectiveness of these systems lies not only in their accuracy but also in their ability to adapt to emerging threats.
One of the key advantages of AI in fraud prevention is its capability to analyze large volumes of data at lightning speed. This allows financial institutions to identify potential fraud cases in real time. Instead of relying on outdated methods, banks can instantly flag suspicious transactions and prevent losses.
Key Advantages of AI in Fraud Prevention
Understanding the specific benefits of AI-driven fraud prevention can assist banks in making smarter decisions. These advantages include:
- Increased Accuracy: AI systems reduce false positives by learning from historical data and customer behaviors.
- Real-Time Monitoring: Continuous transaction analysis ensures immediate detection of anomalies.
- Cost Reduction: Minimizing fraud incidents reduces financial losses and operational costs.
- Enhanced Customer Experience: Faster processing of legitimate transactions leads to higher customer satisfaction.
Another significant benefit is the enhancement of a bank’s reputation. By adopting AI-driven solutions, institutions demonstrate their commitment to protecting customers’ finances. This proactive approach can attract more clients who prioritize security.
In addition, AI can learn from new threats. As fraud tactics evolve, AI technologies continuously update their algorithms, ensuring banks stay ahead of fraudsters. This adaptability makes AI an invaluable asset in the fight against fraud.
How AI algorithms analyze transaction data
How AI algorithms analyze transaction data is crucial for identifying and preventing fraud in banking. These algorithms quickly process vast amounts of information, allowing banks to detect suspicious activities efficiently. By utilizing machine learning techniques, they learn from historical data, which helps improve the accuracy of fraud detection.
The analysis starts when transactions occur. AI systems gather data from various sources, including customer profiles and previous transaction histories. This data is then evaluated against established patterns to find discrepancies.
Key Steps in AI Data Analysis
There are several key steps AI algorithms utilize to analyze transaction data:
- Data Collection: AI collects data from different channels, such as online purchases, ATM withdrawals, and more.
- Pattern Recognition: The system identifies normal transaction behavior for individual users.
- Anomaly Detection: By comparing new transactions with established patterns, AI can spot unusual activities.
- Feedback Loop: AI continuously learns from past data to improve its predictive accuracy.
As transactions occur, algorithms remain on high alert, assessing every detail, from the time of the transaction to the amount. This thorough examination allows banks to flag transactions that deviate from a user’s typical behavior.
Not only do these AI algorithms help prevent fraud, but they also contribute to improving customer experiences. By processing transactions quickly and efficiently, they reduce the chances of legitimate transactions being declined, which can frustrate customers.
With every detection of fraud, these algorithms refine their models, helping banks stay ahead of evolving security threats. Their ability to adapt to new patterns of fraud is essential in maintaining trust and security in financial transactions.
Real-world applications of AI in banks
Real-world applications of AI in banks showcase how technology can transform financial services. Banks are increasingly adopting AI to enhance operational efficiency and security. By implementing AI-driven solutions, they can streamline processes and provide better services to their customers.
One significant application of AI is customer service automation. Many banks now use chatbots that are available 24/7 to answer customer inquiries. This technology allows banks to provide instant support while reducing the workload on human agents, enabling them to focus on more complex issues.
AI in Fraud Detection
Another important application is in fraud detection. Banks use advanced algorithms to analyze patterns and identify suspicious transactions in real time. These systems learn from previous data, continually improving their ability to spot potential threats and protect customer accounts.
- Transaction monitoring: AI systems monitor transactions around the clock for unusual activities.
- Pattern analysis: Algorithms examine behavior trends to create user profiles.
- Instant alerts: Customers receive notifications about potentially fraudulent activities, allowing for quick action.
Moreover, AI is employed in risk management. Banks assess the creditworthiness of borrowers more accurately by analyzing extensive datasets. This leads to better lending decisions and reduces the risk of defaults.
In addition, AI tools help optimize investments. By analyzing market trends and customer preferences, banks can offer personalized investment solutions that align with clients’ financial goals. Automated trading systems also use AI to execute trades at ideal moments, maximizing returns.
Future trends in banking fraud detection
Future trends in banking fraud detection are exciting as technology evolves. With the increasing sophistication of fraud techniques, banks must adopt new strategies to stay ahead. Innovations in artificial intelligence (AI) and machine learning are paving the way for more effective detection methods.
One significant trend is the use of advanced analytics. Banks are beginning to utilize predictive analytics to identify potential fraud before it occurs. By analyzing historical data, they can develop models that predict which accounts are at risk. This proactive approach allows financial institutions to intervene sooner.
AI and Machine Learning Enhancements
Moreover, AI continues to improve with neural networks. These complex systems can recognize patterns within vast amounts of transaction data. As a result, they become better at distinguishing between legitimate and fraudulent activities over time. This leads to:
- Higher accuracy: Enhanced algorithms reduce false positives and negatives.
- Faster detection: AI systems can analyze data at unprecedented speeds.
- Adaptability: Algorithms quickly adjust to new trends in fraud.
Another trend is the integration of biometrics. Banks are increasingly using biometric data, such as fingerprints and facial recognition, to authenticate transactions. This adds a layer of security that is difficult for fraudsters to duplicate.
Moreover, the rise of real-time monitoring systems is changing how banks detect fraud. These systems can analyze transactions as they happen, providing immediate alerts for suspicious activities. This capability minimizes the risk of loss by enabling quick responses to potential threats.
Finally, collaboration among financial institutions is becoming more common. By sharing data and insights about fraud attempts, banks can create a combined defense. This collective intelligence helps them better understand and tackle emerging fraud trends.
FAQ – Frequently Asked Questions about AI in Banking Fraud Detection
How does AI improve fraud detection in banks?
AI enhances fraud detection by analyzing large volumes of transaction data to identify patterns and anomalies, enabling real-time alerts for suspicious activities.
What role do biometric measures play in banking security?
Biometric measures, such as fingerprint and facial recognition, add a layer of security that makes it difficult for unauthorized users to access accounts.
What is predictive analytics and how is it used in banks?
Predictive analytics involves using historical data to forecast potential fraud before it occurs, helping banks to take proactive measures.
Why is collaboration among banks important in combating fraud?
Collaboration allows banks to share insights and data about fraud attempts, leading to a stronger collective defense against emerging threats.